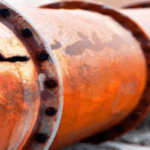
Sulfur Deposition in Natural Gas Transmission Line Joints
مهر ۴, ۱۴۰۳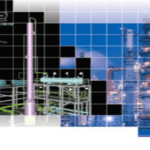
Simulation of Aspen Hysys and Optimization Process
مهر ۴, ۱۴۰۳Introduction
Machine learning has initially been utilized in lithology identification, curve reconstruction of logging data, reservoir parameter estimation, and other logging processes and interpretations, showcasing significant potential. Computer vision proves effective in selecting initial seismic failures, fault identification, and various seismic processing and interpretation tasks.
The application of data mining in drilling, completion, and surface and subsurface engineering has led to the development of smart equipment and integrated software solutions. To harness the potential of artificial intelligence in oil exploration and production, it is essential to focus on intelligent production equipment, automated processing and interpretation, and professional software platforms. Key highlights in the development of digital basins include rapid intelligent imaging logging tools, intelligent seismic node acquisition systems, smart rotary drilling, intelligent fracture technology and equipment, and real-time monitoring and control of regional injection and production processes.
Research and Development of Artificial Intelligence in Oil Companies
Strategies such as open innovation, deep integration of production, training and research, and collaboration with IT companies are being adopted globally by oil companies to make the oil and gas industry smarter. Through collaborative efforts with technology companies, international oil companies have achieved intelligent upstream operations, resulting in cross-alliance formations such as Total + Google Cloud, Chevron + Microsoft, Shell + HP, and others.
Artificial Intelligence in the Oil and Gas Industry
Artificial Intelligence (AI) encompasses a wide range of technologies that enable machines to perform tasks typically requiring human intelligence, such as learning, problem-solving, and decision-making. AI technologies include machine learning, natural language processing, computer vision, and robotics.
AI finds numerous applications across various sectors of the oil and gas industry, including exploration, drilling, production, refining, and distribution. It also plays a significant role in optimizing energy consumption and product quality in refining processes. Additionally, AI is utilized in pipeline monitoring, predictive maintenance, and safety management throughout the distribution phase.
Top 10 Applications of Artificial Intelligence in the Oil and Gas Sector
AI opens doors to numerous diverse applications in oil and gas, expanding the horizons of this sector. Here, we explore the top 10 applications of AI in the oil and gas industry in detail:
-
Demand Forecasting
One prominent application of AI in the oil and gas industry is demand forecasting, which serves as a crucial approach for better business decision-making. AI algorithms leverage vast amounts of historical data and current market trends to generate accurate forecasts for oil and gas product demands.
Furthermore, beyond demand forecasting, the use of AI aids in better planning of logistics and supply chain activities. This underscores the significant impact of AI on the oil and gas industries.
-
Predictive Maintenance
Predictive maintenance is a standout application of AI in oil and gas that enables businesses to adopt a methodical approach. AI-based predictive maintenance systems continuously monitor the health of equipment through sensors and data analytics.
-
Oil Exploration
By enhancing the success rate of discovering sustainable oil reserves, companies can allocate resources more effectively and reduce the financial risks of dry wells. Additionally, AI-driven exploration supports sustainable practices by minimizing environmental impacts through targeted drilling. This highlights the critical role of AI in the oil and gas industries.
-
Price Volatility Management
AI in oil and gas software helps companies analyze real-time market data and historical trends to assess the nature of oil and gas price volatility. Predictive models can forecast price movements, enabling businesses to make informed pricing strategies, hedge risks, and inventory management decisions.
-
Safety Enhancements
Safety is paramount in the oil and gas industry, and AI systems play a crucial role in bolstering it. AI can identify potential hazards and generate early warnings through continuous monitoring of operations and analysis of data from various sources. This allows for immediate corrective actions, preventing accidents and ensuring a safer work environment.
Additionally, AI solutions for oil and gas can analyze incident data to identify patterns and implement preventive measures, thereby reducing the risk of future incidents.
-
Robotic Process Automation (RPA) for Back Office Tasks
RPA streamlines back-office operations by automating repetitive and time-consuming tasks such as data entry, invoice processing, and report generation. Employees are freed from mundane tasks, allowing them to focus on more strategic and value-added activities.
Furthermore, RPA enhances data integrity and compliance by ensuring consistency and reducing the likelihood of human error. By integrating AI for oil and gas, companies can further optimize their operations, leveraging advanced analytics and predictive maintenance to boost productivity and innovation.
-
Detection and Response to Oil and Hydrocarbon Leaks
Utilizing advanced sensors and satellite imagery, the application of AI in oil and gas software enhances the detection and response to oil spills and hydrocarbon leaks. These systems can quickly identify and assess the extent of leaks, enabling rapid response measures to contain and mitigate environmental damage.
AI also facilitates real-time monitoring and predictive analytics, helping companies manage and prevent leaks proactively. This protects the environment and reduces cleanup costs and potential liabilities.
-
Regulatory Compliance
Ensuring compliance with regulations in the oil and gas industry is vital, and the use of AI in oil and gas solutions assists in this by continuously monitoring operations against established standards. AI can analyze vast volumes of data to identify compliance issues in real-time, enabling companies to address them promptly.
This proactive approach minimizes the risk of non-compliance penalties and enhances the company’s reputation. Moreover, AI solutions for oil facilitate streamlined reporting processes, ensuring accurate and timely submissions to regulatory bodies.
-
AI-Driven Supply Chain Management
AI optimizes supply chain management in the oil and gas industry by providing insights into demand forecasting, inventory management, and logistics planning. Predictive analytics can forecast demand fluctuations, allowing companies to adjust their supply chain operations accordingly. This results in improved efficiency, reduced costs, and enhanced customer satisfaction.
AI also aids in identifying potential disruptions in the supply chain, enabling companies to implement contingency plans and maintain operational continuity. Enhanced visibility and agility in the supply chain ultimately contribute to a more resilient and responsive supply chain network.
-
Advanced Reservoir Characterization Modeling
In the oil and gas industry, advanced reservoir characterization and modeling based on AI have transformed how companies manage and optimize their reservoirs. This approach integrates vast amounts of data from seismic surveys, well logs, and production history using advanced AI techniques to provide an accurate and comprehensive representation of reservoirs.
Real-World Examples of Artificial Intelligence in the Oil and Gas Industry
Leading companies are pioneering the use of artificial intelligence (AI) in oil and gas exploration to overcome complex challenges, drive innovation, and set new industry standards. Here are some key players spearheading this technological transformation:
Royal Dutch Shell
Shell enhances predictive maintenance in the oil and gas industry by deploying advanced machine learning, significantly reducing downtime and maintenance costs. Additionally, AI-based seismic data analysis enables more accurate and efficient exploration while advancing innovations in drilling operations and supply chain logistics.
British Petroleum (BP)
British Petroleum utilizes AI to enhance its operational efficiency and cost-effectiveness. Their use of AI in analyzing geological data simplifies the identification of potential drilling locations, ensuring higher accuracy and better resource allocation. Furthermore, BP’s AI solutions optimize production processes and energy management, reflecting their commitment to technological advancement.
ExxonMobil
ExxonMobil leverages AI to transform reservoir management, predictive maintenance, and safety monitoring. They simulate reservoir behavior using AI to maximize extraction efficiency and recovery rates.
Chevron
Chevron integrates AI into its oil and gas operations to enhance exploration and production activities. They process seismic data with unprecedented accuracy using machine learning algorithms, improving subsurface imaging and oil reservoir identification.
Beneficial Role of AI in Advancing the Oil and Gas Sector
In this section, we explore the numerous benefits AI brings to the oil and gas industry. From increased operational efficiency to improved safety and better decision-making, AI transforms various aspects of this sector. These advantages lead to increased productivity, cost reduction, and competitive advantage in the market.
-
Better Decision Making
- From exploration to production and distribution, AI-driven insights help companies optimize their operations. Better decision-making leads to improved efficiency and cost savings. Additionally, AI aids in identifying new opportunities and mitigating risks.
-
Cost Reduction
- AI-driven productivity significantly reduces operational costs. Predictive maintenance minimizes downtime and repair costs, while optimized production plans reduce waste. AI also enhances supply chain management, leading to lower inventory and logistics costs.
-
Environmental Protection
- AI assists in the rapid identification of oil spills and hydrocarbon leaks by analyzing sensor data and satellite images. Quick detection enables prompt responses, minimizing environmental impacts and reducing cleanup costs. AI-driven environmental management supports sustainable practices in the industry.
-
Competitive Advantage
- Companies adopting AI gain a significant competitive edge by being more efficient, responsive, and innovative. AI-based insights and optimizations help businesses stay ahead of competitors. Increased operational productivity and cost savings result in better financial performance. Moreover, AI supports the development of new products and services, fostering innovation and market differentiation.
-
Enhanced Customer Experience
- AI improves customer interactions by delivering personalized experiences and timely responses. Advanced data analytics enable companies to better understand customer needs and preferences, leading to improved service quality and higher customer satisfaction.
-
Innovation and Continuous Improvement
- AI fosters a culture of innovation and continuous improvement by enabling companies to analyze data and identify areas for enhancement. AI drives ongoing process and operational optimization by pinpointing inefficiencies and suggesting improvements. This continuous enhancement leads to the development of new technologies and solutions, ensuring companies remain at the forefront of industry advancements.
Applications of AI in Oil Exploration and Development
Logging Technology
Since its inception in 1927, logging technology has experienced over 90 years of advancement, evolving from analog logging to digital logging, direct digital logging, imaging logging, and now entering the era of intelligent logging.
Data Collection Logging
Foreign companies have commercialized their products in data collection and remote logging, such as Schlumberger’s remote logging center, smart structure tester, and Techlog well software capable of intelligent processing and interpretation. Eleven data server centers and 14 remote logging centers are located worldwide, staffed by 108 operational engineers who facilitate remote collaborative work and expert decision-making. Twenty percent of logging operations are completed by remote logging centers, amounting to over ten thousand jobs.
Processing and Interpretation of Logging Data
Logging data is characterized by large volumes and heterogeneity from multiple sources, posing significant challenges for interpretation specialists, such as multiple solutions and uncertainties that complicate the identification of pay zones. Consequently, leveraging AI and other technologies to enhance work efficiency and reduce interpretation error rates is essential. In recent years, AI applications in logging interpretation have mainly focused on automatic depth correction, automated report generation, intelligent classification, curve reconstruction, lithology identification, image report interpretation, reservoir parameter estimation, potential oil and gas evaluation, and shear wave velocity prediction.
Integrated Software
Techlog well software comprises a suite of intelligent functional modules, including analysis, prediction, and classification of curve-sensitive factors, and curve reconstruction, significantly supporting intelligent interpretation. In China, application platforms like the CNPC Dream Cloud Collaboration Platform, integrated LEAD report processing and interpretation software, and the new generation of multi-well structural assessment software CIFlog have been developed. AI applications in reservoir description and simulation, as well as in multi-well reporting interpretation, have achieved preliminary results, and a geo-steering system for horizontal drilling is in development.
Directions for Developing Artificial Intelligence Programs
Artificial intelligence (AI) technology is certainly providing a new impetus for scientific advancements across the entire oil and gas industrial chain. Considering the demand for Exploration & Development (E&D) in oil and the research status on AI technology, future development directions will cover three main aspects:
Intelligent Equipment
Oil companies are increasingly utilizing robots instead of humans for hazardous operations. So far, robots have been successfully employed in pipeline surveillance, deep-water operations, and other high-risk tasks.
Drone technology is gradually being utilized in E&D operations, especially in geophysical exploration, where drones can perform geological surveys, data collection, video monitoring, material delivery, engineering rescue, and more. Meanwhile, thanks to the integration of specialized software into equipment, tools are becoming increasingly intelligent.
Automatic Data Processing and Interpretation
The application of data mining and mathematical statistics in oil E&D has proven quite successful, as evidenced by its extensive use in logging curve interpretation, reservoir parameter prediction, and other tasks. In recent years, with the continuous advancement of deep learning, ensemble learning, transfer learning, and other technologies, AI’s superiority in processing, analyzing, and predicting data has become apparent. Deep learning, ensemble learning, transfer learning, and strong memory learning technologies are expected to find profound applications in the future for the automatic processing and analysis of petrophysical data, seismic images, logging curves, digital cores, production operations, and other data.
Professional Software Platforms
In terms of oil E&D, professional software and information systems are the vehicles and core technologies of AI. Professional software is the most crucial tool for research, a fundamental achievement of expert knowledge, and a primary competitive advantage for oil companies and service firms.
Reservoir Modeling
Uncertainty in reservoir exploitation is significant when trying to understand how a solid rock formation responds to induced hydraulic fracturing treatments. Proper utilization of complex or high-dimensional reservoir data through AI can better quantify uncertainty. For instance, it can optimize fracture spacing and design models.
The power of pattern recognition through AI enables such speed in historical fitting. Modeling software is a composite system that combines artificial neural networks, fuzzy set theory, and genetic algorithms.
For instance, reservoir management software operates in three stages: data mining, data-driven modeling, and model analysis. First, data, often high-dimensional, is pre-processed for quality control and extracted to discover hidden and potentially useful patterns.
The first stage continues with identifying key performance indicators (KPIs), where the software determines parameters that control outcomes. Relevant KPIs vary from one field to another, and there can be hundreds of indicators to examine in a specific project.
In the second stage, data is processed into a machine-readable format that presents the reservoir model. This model is developed based on field measurement data, interpreted either quantitatively or without engagement.
Enhancement of Chemical Processes with AI
Predicting Molecular Properties
Predicting molecular properties is a significant challenge in computer-aided molecular design, and deep learning models are excellent for predicting molecular properties, which can greatly accelerate experimental studies. Two main types of models stand out in predicting molecular properties: graph neural networks and sequence-based neural networks, which differ in representing various molecules. The former requires graphical molecular information, while the latter requires a string representation of molecular structures.
The direct use of matrices to capture molecular structure information is a common method for molecular representation known as molecular graphs, which can be trained using graph neural networks.
Molecular Design
Computer-aided molecular design is another important research direction in cheminformatics, and designing suitable molecules based on requirements has been a dream task for chemists. Similar to predicting molecular properties, both graph generation models and text generation models in deep learning can be utilized for molecular design.
Conclusion
The application of AI in the oil E&D sector is newly on the right track and has not yet produced disruptive achievements, but it has shown high potential. The results presented can be summarized in two aspects: first, the primary application of intelligent equipment. Drones and robots are used to replace humans for monitoring, covering pipelines, unattended platforms, and more. Second, the use of big data, machine learning, and other IT technologies in data processing and analysis for exploration and development.